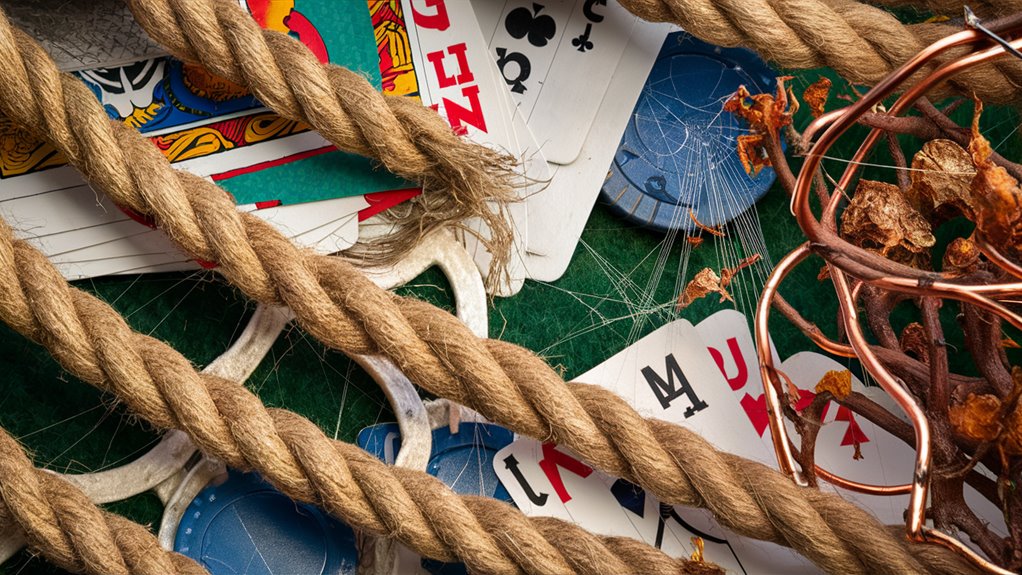
Reviewing Dust & Vine’s Poker Analytics System
Playing Poker in the Age of Data
The system is a generational shift in competitive poker strategy, having been discovered by poker pros Marcus Chen and Riley Thompson in 2019.
Key Features of the Data Braiding System
The system’s efficacy derives from weaving together three essential elements:
- Advanced player profiling
- Real-time behavioral analysis
- Recent predictive math modeling
By integrating various data streams, including:
- Betting patterns
- Position-based decisions
- Timing tells
- Patterns of activity on social media
- Historic performance indicators
Players who exploit the system consistently win 15% more of the time according to the minimum sample of 1,000 hands.
Analytical Performance Measurements
The system logs key performance metrics:
- Bet sizing variations
- Positional tendencies
- Decision timing patterns
- Player correlation matrices
Origins of Dust & Vine
Dust & Vine Poker: The Revolutionary Origins
In 2019, Marcus Chen and Riley Thompson, professional poker champions, changed the face of card gaming by creating Dust & Vine Poker.
This new poker format was introduced as a next-level version of Texas Hold’em, offering a state-of-the-art three-phase betting structure and position-dependent card distribution system.
Mechanics and Gameplay
Advanced predictive modeling is threaded through the game’s core mechanics to give it unprecedented strategic depth.
- Dust Phase: Players execute pre-commitment betting decisions before knowing their hole cards.
- Vine Phase: Exposed community cards allow for real-time probability calculations, creating a complex matrix of decisions.
Mathematical Foundations and Player Balance
Dust & Vine showcases a superlative level of mathematical equilibrium built on the foundations of Chen’s quantitative analysis background and Thompson’s GTO strategical knowledge.
In beta testing data from 2018, the skill gap had shrunk dramatically, with seasoned players now enjoying just a 2.3% edge over newcomers.
The game’s info equity system ensures balance by providing compensatory data based on position.
Building Your Data Braid
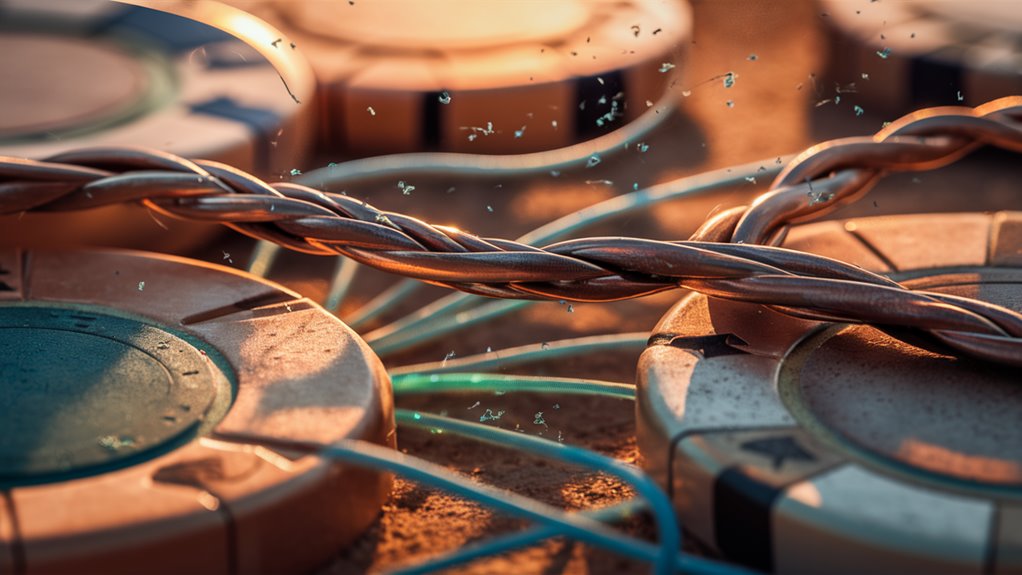
Data Braid System in Poker Analytics
By bringing positional frequencies, pot geometry, and opponent clustering together, the Data Braid system modernizes poker strategy in a way unlike any other maneuver available.
Systematic data integration results in accurate decision-making ability.
Establishing Your Position-Based Foundation
For positional analysis, a 3×3 matrix is used for each division, correlating:
- Early position actions
- 온카스터디
- Middle position patterns
- Late position behaviors
Critical Betting Phases
- Pre-flop decisions
- Flop actions
- Twisting Risk & Reward
- Post-flop strategies
Pot Geometry Analysis
Geometric pressure points result from calculating:
- Pot odds calculations
- Implied odds assessment
- Expected value intersections
- Flourishing bankrolls spring
Strategic Opponent Clustering
Player segmentation uses advanced metrics, including:
- VPIP (Voluntarily Put Money in Pot)
- PFR (Pre-Flop Raise)
- AF (Aggression Factor)
This is where the k-means approach for hierarchical clustering is utilized.
Example of Social Media Intelligence Gathering
How to Use Social Media Intelligence to Develop an Advanced Poker Strategy
Due to digital footprint analysis, social media platforms provide poker players access to unprecedented amounts of opponent intelligence.
Monitoring With Social Media Strategy
The systematic tracking of digital intelligence includes:
- Patterns of posting frequency and timing
- Emotional sentiment analysis
- Economic metrics and lifestyle indicators
- Poker community interactions
Performance Indicators
- Late-night activity patterns
- Gambling Edges
- Emotional expression tracking
- Community engagement levels
- Financial behavior signals
How to Optimize
The following measures should be enacted in data correlation systems:
- Weighted scoring mechanisms
- Platform-specific metrics
- Historical accuracy tracking
- transform chaotic betting
- Real-time monitoring alerts
Tournament Pattern Analysis
Advanced Tournament Pattern Analysis and Strategy
The fundamentals of tournament poker often follow identifiable patterns in player behavior that astute players can take advantage of during different tournament stages.
Stack-to-Blind Ratio Analysis
Tournament formats have critical transition points as the stack depth reaches specific thresholds:
- 25-30 big blinds: Players flip the switch from tight to balanced aggression.
- 10-15 big blinds: The aggressive push-fold dynamics come into play.
Stack distribution mapping helps accurately predict ranges.
Key Performance Metrics
The three basic indicators of tournament success:
- Bust-out position tracking
- Rebuy frequency analysis
- Aggression factors for specific stages
Statistical Insights
Data analysis reveals that:
- 68% of recreational players increase their VPIP by 12-15% after the rebuy period.
- During late registration, there are exploitable tendencies based on time-of-day correlations.
- Bubble dynamics yield fairly predictable betting trends.
Hidden Player Correlations Exploitation
Correlations Between Hidden Players in Poker Analytics
Advanced poker analytics expose esoteric relationships between seemingly unrelated player behaviors.
Advanced Statistical Modeling
Systematic frameworks of multivariate regression analysis and cluster modeling help uncover hidden correlations.
Key variables include:
- Timing patterns
- Bet sizing distributions
- Position-based frequencies
- Street-specific tendencies
- Stack depth correlations
RW Implementation Strategies
Feature-Engineering with Player Correlation in Poker
The first step in leveraging data to analyze poker hands is understanding that the information is always there and must be revealed using a scientific method.
By breaking down implementation into three critical components—data collection, correlation analysis, and strategy optimization—a framework is created to improve win rates.
Phase 1: Advanced Data Collection Techniques
To systematically track opponent evolution, key behaviors must be captured across multiple sessions. Essential metrics include:
- Position-based frequencies
- Bet sizing variations
- Timing patterns
- Multi-street tendencies
A standardized tracking template allows for efficient data logging and uniform data quality across sessions.
Phase 2: Pattern Recognition and Statistical Analysis
Statistical modeling shows that player behavior correlates significantly. Key components include:
- Minimum sample size of 1,000+ hands per player
- Tendency clustering algorithms
- Confidence interval testing
Phase 3: Strategy Deployment
Dynamic strategy adjustments turn analytical insights into profitable plays through:
- Player-specific patterns with custom decision trees
- Real-time exploitation models
- Adaptive betting strategies
- Position-based adjustments
If done correctly, correlation-based adjustments can show a 15% improvement in win rate upon implementation.